The Academic's Guide to AI: Enhancing Research and Learning
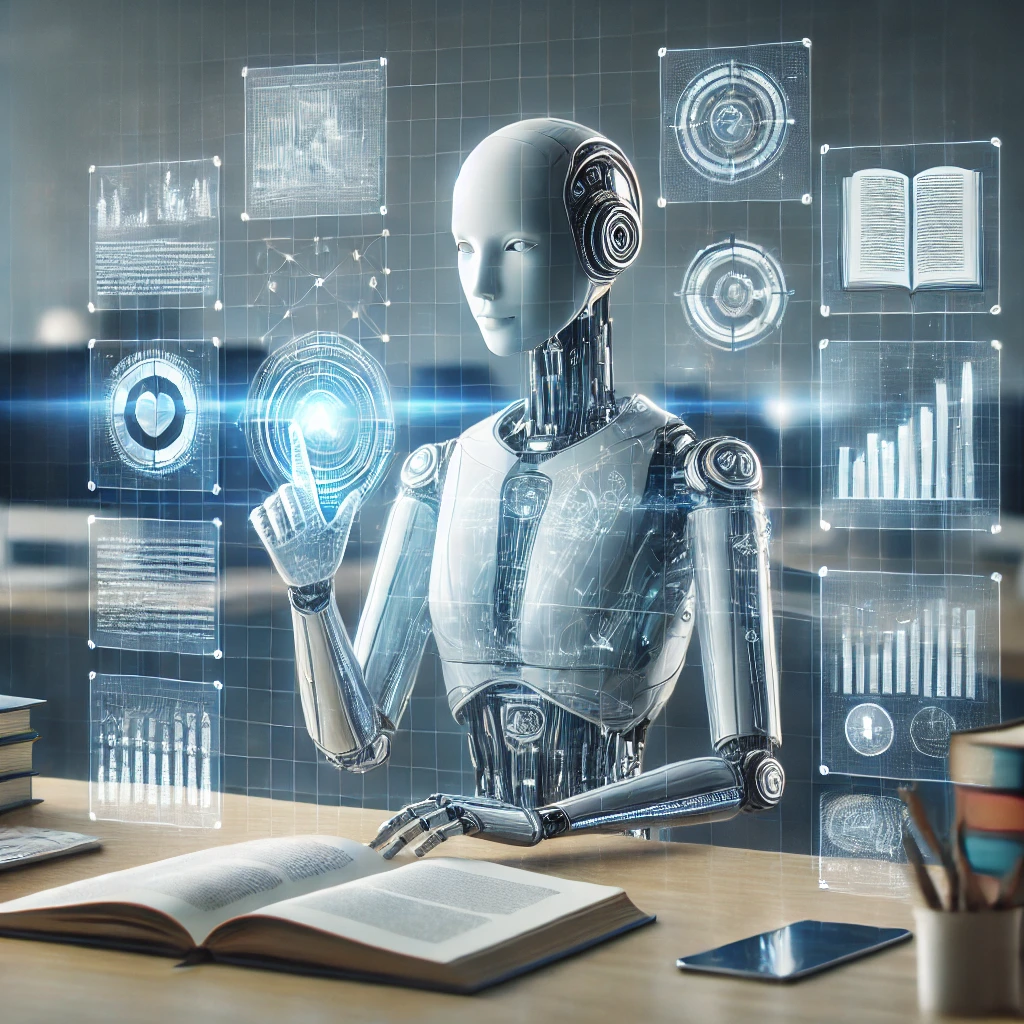
The Academic’s Guide to AI: Enhancing Research and Learning
Artificial intelligence has quietly but decisively entered the halls of academia. As AI tools become increasingly sophisticated, researchers and educators across disciplines find themselves at a crossroads: How can we harness these powerful tools while maintaining academic integrity and enhancing rather than diminishing the quality of our work?
The answer lies not in whether to use AI, but in understanding how to use it effectively. The integration of AI into academic work represents a significant shift in how we approach research, analysis, and learning. Much like the introduction of computers and the internet transformed academic work decades ago, AI tools are opening new possibilities while raising important questions about proper usage and methodology.
The key principle that should guide any academic use of AI is enhancement rather than replacement. These tools should augment our capabilities, sharpen our analysis, and free up time for deeper thinking—not substitute for critical thought or original research. When used thoughtfully, AI can serve as a powerful research assistant, helping to process vast amounts of information, identify patterns, and generate new perspectives while leaving the crucial work of analysis and interpretation to the researcher.
Consider how this plays out across different fields. Historians can use AI to analyze vast collections of primary documents, identifying patterns and connections that might take years to discover manually. Scientists can quickly summarize and cross-reference research papers, spotting methodological trends and potential innovations. Sociologists can process large datasets of survey responses, while literature scholars can identify themes and patterns across extensive texts.
The current landscape of academic AI tools offers various options, each with its own strengths. ChatGPT has become widely known for its general capabilities and ability to engage in sophisticated dialogue, though it lacks direct source attribution. Claude stands out for its built-in ethical principles and strong analytical capabilities. Perplexity offers valuable source attribution and academic-focused features.
Deep Dive: NotebookLM as a Scholar’s Assistant
Among the various AI tools available to academics, Google’s NotebookLM stands out as a particularly sophisticated solution designed specifically for scholarly work. Unlike general-purpose AI chatbots, NotebookLM operates on a fundamentally different principle: it works directly with your source materials, creating a controlled environment for academic analysis.
What sets NotebookLM apart is its source-based approach. Rather than drawing from a vast, unspecified knowledge base, NotebookLM analyzes only the documents you provide. This focused approach offers several crucial advantages for academic work. When you upload research papers, historical documents, or any scholarly text, the tool creates a dedicated “notebook” that becomes your digital research assistant for that specific material.
The tool’s citation system is particularly impressive. Every insight or analysis it provides comes with direct references to the source material, including specific page numbers or sections. This feature alone transforms how researchers can interact with complex texts. Imagine analyzing Kant’s “Critique of Pure Reason” or Darwin’s “Origin of Species” – NotebookLM can help you track concepts across hundreds of pages while maintaining precise source attribution.
Another powerful feature is its ability to handle multiple related documents simultaneously. Researchers can upload several papers on a similar topic and ask NotebookLM to identify patterns, commonalities, and contradictions across the texts. This capability is invaluable for literature reviews or when trying to synthesize findings from multiple studies.
The Art of Academic AI Prompting
Success with academic AI tools, particularly NotebookLM, depends heavily on how you frame your queries. The art of “academic prompting” deserves special attention as it differs significantly from casual AI interaction. Here are the key principles for effective academic AI prompting:
Query Construction
- Start broad, then narrow down
- Use academic terminology
- Specify the type of analysis needed (comparative, analytical, synthetic)
- Request specific citations or examples
Follow-up Refinement
- Build on previous responses
- Ask for clarification of specific points
- Request additional context or opposing viewpoints
- Verify interpretations against source material
The difference between a basic prompt and an effective academic prompt can be substantial. Instead of asking “What does this paper say about climate change?”, a better approach would be “Analyze the methodology used to measure climate impacts in the results section, with particular attention to statistical significance and potential limitations.”
Integrating AI into Research Workflow
One of the most powerful ways to leverage tools like NotebookLM is to integrate them directly into your research workflow from the beginning of a project. Start by creating dedicated notebooks for different aspects of your research – one for literature review, another for methodology analysis, and perhaps another for theoretical framework development.
When approaching a new research topic, consider this systematic approach:
- Upload key papers and create an initial notebook
- Ask NotebookLM to identify major themes and methodological approaches
- Create concept maps based on the AI’s analysis
- Verify and expand upon the AI’s insights through traditional research methods
- Document both AI-assisted discoveries and your own analysis
The key is to maintain a balance between AI assistance and independent scholarly work. Use NotebookLM’s insights as a starting point for deeper investigation, not as final conclusions. The tool should prompt questions, suggest connections, and highlight areas for further study – all while maintaining a clear trail of citations and sources.
The practical application of AI in academic work requires thoughtful implementation. Rather than asking AI to write papers or draw conclusions, researchers can use it more effectively as a research assistant. For example, when beginning a literature review, AI can help identify key themes across papers, suggest connections between different theoretical frameworks, or highlight methodological patterns. The researcher then investigates these suggestions, verifies them, and develops their own analysis.
Data analysis presents another valuable use case. While AI shouldn’t be trusted to draw final conclusions, it can help identify initial patterns and potential areas for investigation. A sociologist analyzing survey responses might use AI to spot preliminary trends, which they then investigate more thoroughly using traditional research methods.
One of the most valuable applications of AI in academic work is in the early stages of research. When facing a new topic or field, AI can help researchers get oriented quickly, suggesting key papers, identifying major debates, and highlighting crucial concepts. This initial orientation can make subsequent deep reading and analysis more focused and productive.
The future of AI in academia looks promising, with tools becoming more specialized and better integrated with research workflows. However, the key to successful integration lies in maintaining clear boundaries and ethical guidelines. Individual researchers and institutions need to develop clear policies about AI usage, ensuring that it enhances rather than compromises academic integrity.
Perhaps most importantly, academics need to maintain transparency about their AI use. Just as we cite human colleagues who provide significant input to our work, we should acknowledge when AI tools have contributed to our research process. This transparency helps maintain academic integrity while advancing our understanding of how to best utilize these tools.
This combination of AI capabilities with traditional academic rigor represents the future of scholarly work. By understanding and appropriately utilizing tools like NotebookLM, researchers can enhance their analytical capabilities while maintaining the highest standards of academic integrity. The goal isn’t to revolutionize academic work, but to evolve it thoughtfully, maintaining its core principles while embracing new possibilities for discovery and analysis.
Note: When using AI tools in academic work, always verify your institution’s specific guidelines and policies regarding AI usage in research and writing.
Details
11 Nov 2024
Category: Academic Research, AI Technology, Research Tools